Speakers
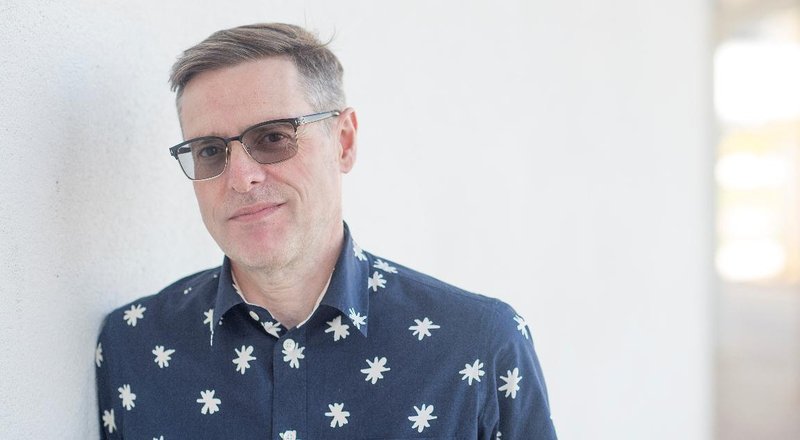
Prof. Mark Girolami, The Alan Turing Institute and University of Cambridge
Inaugural talk on "Engineering the Future and the Future of Engineering"
We face a number of global challenges and engineering is at the core to ‘engineering our future’ as highlighted by Lord Patrick Vallance who has said "There’s no doubt that engineering has a major central role to play in the global challenges, whichever ones you choose to pick”. The question is what is the future of engineering to ensure we are effective in addressing these big questions? Is it AI and related data driven technologies? Is this future of engineering a data centric, and what would this actually mean?
Professor Mark Girolami is the Chief Scientist of The Alan Turing Institute, the UK’s National Institute for data science and artificial intelligence and took up this role in October 2021. He was one of the original founding Executive Directors of the Institute and previous to his role as Chief Scientist he led the Turing’s Data-centric Engineering programme, which is principally funded by Lloyd’s Registry Foundation. In 2019, Mark was elected to the Sir Kirby Laing Professorship of Civil Engineering at the University of Cambridge where he also holds the Royal Academy of Engineering Research Chair in Data Centric Engineering. He is a fellow of Christ’s College Cambridge. Prior to joining the University of Cambridge, Mark held the Chair of Statistics in the Department of Mathematics at Imperial College London. He is an elected fellow of the Royal Academy of Engineering and the Royal Society of Edinburgh.
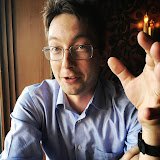
Prof. Steve Brunton, University of Washington
Machine Learning for Scientific Discovery, with Examples in Fluid Mechanics
This work will discuss several key challenges and opportunities in the use of machine learning for nonlinear system identification. In particular, I will describe how machine learning may be used to develop accurate and efficient nonlinear dynamical systems models for complex natural and engineered systems. I will emphasize the need for interpretable and generalizable data-driven models, such as the sparse identification of nonlinear dynamics (SINDy) algorithm, which identifies a minimal dynamical system model that balances model complexity with accuracy, avoiding overfitting. I will also introduce several key benchmark problems in dynamical systems and fluid dynamics that provide a diversity of metrics to assess modern system identification techniques. Because fluid dynamics is central to transportation, health, and defense systems, we will emphasize the importance of machine learning solutions that are interpretable, explainable, generalizable, and that respect known physics.
Dr. Steven L. Brunton is a Professor of Mechanical Engineering at the University of Washington. He is also Adjunct Professor of Applied Mathematics, Aeronautics and astronautics, and Computer science, and he is also a Data Science Fellow at the eScience Institute. He is Director of the AI Center for Dynamics and Control (ACDC) at UW and is Associate Director for the NSF AI Institute in Dynamic Systems. Steve received the B.S. in mathematics from Caltech in 2006 and the Ph.D. in mechanical and aerospace engineering from Princeton in 2012. His research combines machine learning with dynamical systems to model and control systems in fluid dynamics, biolocomotion, optics, energy systems, and manufacturing. He received the Army and Air Force Young Investigator Program (YIP) awards and the Presidential Early Career Award for Scientists and Engineers (PECASE). Steve is also passionate about teaching math to engineers as co-author of four textbooks and through his popular YouTube channel, under the moniker “eigensteve”.
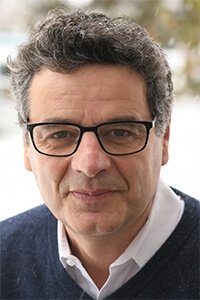
Prof. Petros Koumoutsakos, Herbert S. Winokur, Jr. Professor of Computing in Science and Engineering and Area Chair of Applied Mathematics, Harvard
AI and Scientific Computing: there is plenty of room in the middle
Over the last thirty years we have experienced more than a billion-fold increase in hardware capabilities and a dizzying pace of acquiring and transmitting massive amounts of data. Scientific Computing and Artificial Intelligence (AI) have been key beneficiaries of these advances. In this talk I would outline the need for bridging the decades long advances in Scientific Computing with those of AI. I will use examples from fluid mechanics to argue for forming alloys of AI and simulations for their prediction and control. I will present novel algorithms for learning the Effective Dynamics (LED) of complex systems using machine learning and diffusion models. I will present a fusion of multi- agent reinforcement learning and scientific computing (SciMARL) for modeling and control of turbulent flows. I will also show our recent work on Optimizing a Discrete Loss (ODIL) that outperforms popular techniques such as PINNs by several orders of magnitude. I will juxtapose successes and failures and argue that the proper fusion of scientific computing and AI expertise are essential to advance scientific frontiers.
Petros Koumoutsakos is the Herbert S. Winokur, Jr. Professor of Computing in Science and Engineering and Area Chair of Applied Mathematics at Harvard John A. Paulson School of Engineering and Applied Sciences (SEAS). He studied Naval Architecture (Diploma-NTU of Athens, M.Eng.-U. of Michigan), Aeronautics and Applied Mathematics (PhD-Caltech). He has conducted post-doctoral studies at the Center for Parallel Computing at Caltech and at the Center for Turbulent Research at Stanford University and NASA Ames. He has served as the Chair of Computational Science at ETHZ Zurich (1997-2020). Petros is elected Fellow of the American Society of Mechanical Engineers (ASME), the American Physical Society (APS), the Society of Industrial and Applied Mathematics (SIAM). He is recipient of the Advanced Investigator Award by the European Research Council and the ACM Gordon Bell prize in Supercomputing. He is elected International Member to the US National Academy of Engineering (NAE).
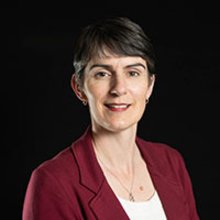
Prof. Beverley McKeon, Professor of Mechanical Engineering and member of the Center for Turbulence Research (CTR), Stanford University
Information from Data: eliciting underlying physics from data-driven studies of turbulence
The availability of data characterizing aspects of complex flow problems in fluid mechanics has advanced radically over the course of the last few decades. What understanding obtained from limited and sparse measurements can or should be retained, and what has been superseded? In this talk, I will review the evolution of methods to obtain information from a range of datatypes, with reference to turbulent and unsteady flows associated with specific scientific questions and practical applications. Some unique capabilities afforded by machine learning will be discussed, along with the gains afforded (or not) by including pre-existing physical understanding.
Beverley McKeon is Professor of Mechanical Engineering and member of the Center for Turbulence Research (CTR) at Stanford University. She was formerly the Theodore von Karman Professor of Aeronautics at the Graduate Aerospace Laboratories at Caltech (GALCIT) and Deputy Chair of the Division of Engineering and Applied Science. She received M.A. and M.Eng. degrees from the University of Cambridge and a Ph.D. in Mechanical and Aerospace Engineering from Princeton University. Her research interests include interdisciplinary, equation- and data-driven approaches to modeling and manipulation of turbulent flows, fundamental experimental investigations of wall turbulence at high Reynolds number, and assimilation of experimental data for efficient low-order flow modeling. McKeon was the recipient of a Vannevar Bush Faculty Fellowship from the DoD in 2017, a Presidential Early Career Award (PECASE) in 2009 and an NSF CAREER Award in 2008, and is a Fellow of the APS and AIAA. She currently serves as co-Lead Editor of Phys. Rev. Fluids and on the editorial board of the Annual Review of Fluid Mechanics. She is Past Chair of the US National Committee on Theoretical and Applied Mechanics.